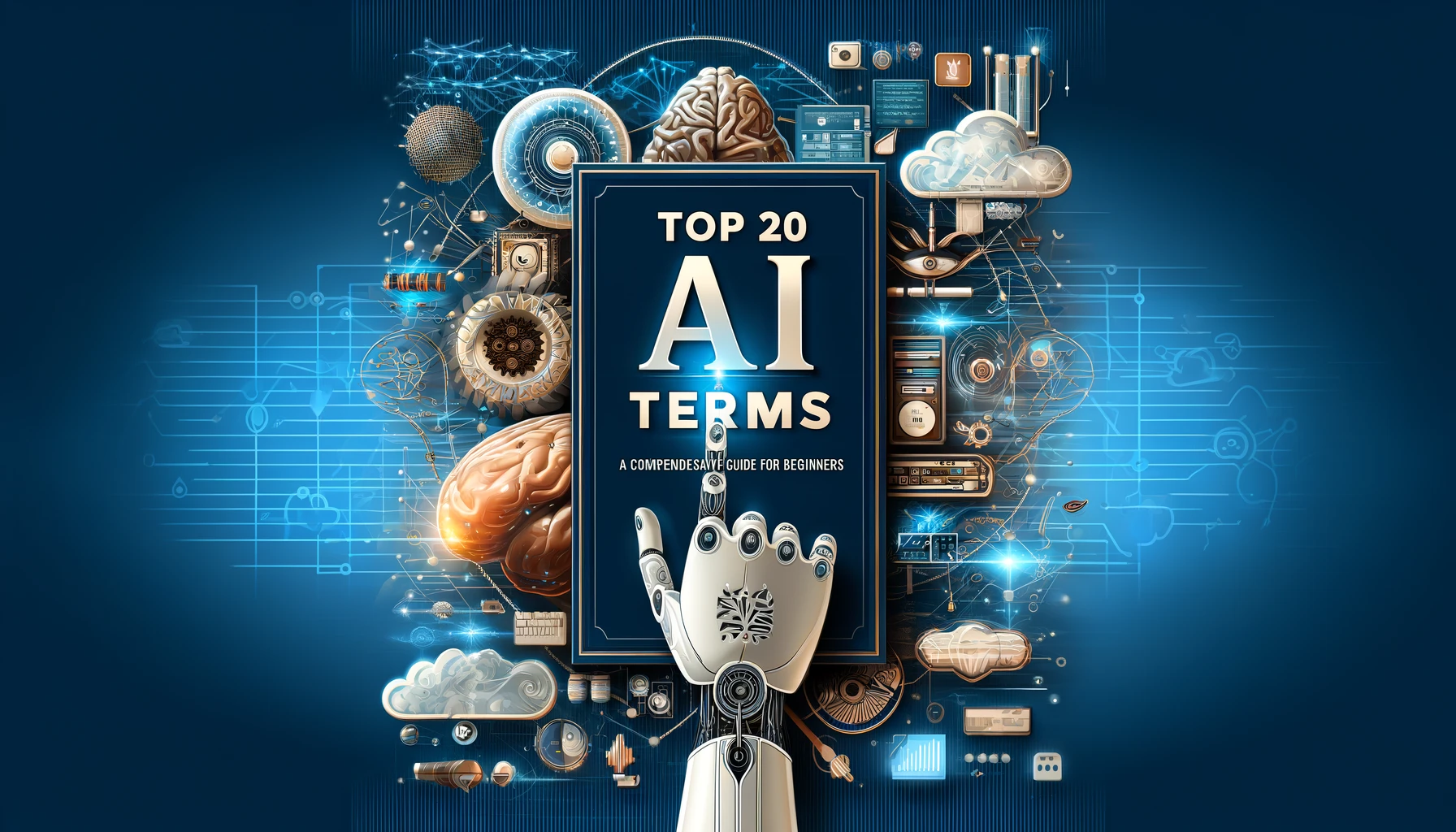
Top 20 AI Terms: A Comprehensive Guide for Beginners
Introduction to AI Terms
AI terms can often sound like buzzwords. However, they carry important meaning and impact. For instance, understanding these terms can help you navigate discussions about technology more effectively. Moreover, knowing these terms can also enhance your ability to implement generative AI solutions in various fields. Here’s a breakdown of the top 20 AI terms you need to know.
Why Is This AI Terms List?
Many people ask about these terms, which seem obvious to those in the field. However, for newcomers and even some seasoned professionals, understanding these concepts can be challenging. That’s why we’ve created this AI cheat sheet. It simplifies complex jargon into easy-to-understand explanations. By familiarizing yourself with these terms, you’ll have a better grasp of AI and its applications. These are terms everyone should know, as they are foundational to navigating and understanding the world of artificial intelligence.
1. Artificial Intelligence (AI)
AI is not just a sci-fi concept. Instead, it serves as the backbone of smart machines performing tasks that typically require human intelligence. Through the integration of classic AI, these machines can carry out complex functions, such as recognizing speech, making decisions, and solving problems, all with remarkable efficiency. This transformative technology enhances our daily lives and contributes to advancements in various industries.
2. Machine Learning (ML)
Machine learning, a key subset of AI, involves teaching systems to learn from data. By analyzing and interpreting large sets of information, these systems can identify patterns and make informed decisions. This process enables machines to improve their performance over time without being explicitly programmed for specific tasks. As a result, machine learning use cases are increasingly prevalent in various fields, from healthcare to finance, enhancing efficiency and accuracy.
3. Deep Learning
Deep learning goes beyond basic machine learning by using neural networks to examine extensive data layers. These networks work like the human brain, processing information through connected layers. This approach allows deep learning to find detailed patterns and insights from complex data, making it a valuable asset in many AI applications. Imagine a system that can understand and predict customer preferences, diagnose medical conditions, or even drive cars by recognizing and reacting to the environment. By simplifying and improving these tasks, deep learning helps businesses and researchers tackle challenges that were once considered impossible.
4. Neural Networks
Neural networks are brain-inspired systems that mimic the way our brains work. They process information through interconnected units, which function similarly to neurons in the human brain. These networks allow computers to recognize patterns, learn from data, and make decisions based on that information. By linking these units together, neural networks can analyze complex data sets and perform tasks that were once thought to be exclusive to human intelligence. This interconnected structure is what makes neural networks so powerful in various applications, from image recognition to language processing.
5. Generative AI
Generative AI represents the imaginative part of artificial intelligence. It can craft new content like artwork or music by studying existing data. For example, these AI models learn patterns and styles from their training and then use this knowledge to create original pieces. Imagine an AI composing a new song or designing a unique visual – it uses what it has learned to produce something fresh and creative. Generative AI, therefore, acts as a digital artist, bringing new ideas and creations to life from the information it has absorbed.
6. Large Language Models (LLMs)
Large Language Models (LLMs), such as GPT (Generative Pre-trained Transformer), are AI models trained on vast amounts of text. These models generate human-like language, making them highly effective for various applications. For instance, LLMs are useful for AI chatbots to create more natural and engaging conversations. They can also assist in content creation by drafting articles, answering questions, and providing detailed explanations. This capability to understand and produce coherent language sets LLMs apart in the field of AI. As these models continue to evolve, their ability to mimic human language improves, offering even more potential uses.
7. Transformer Models
Transformer models are at the core of LLMs. These models excel at handling sequential data, such as language, in an efficient manner. By processing data in parallel rather than sequentially, transformers can manage large volumes of text swiftly and accurately. This capability makes them highly effective for tasks like translation and text generation.
8. Fine-Tuning
Fine-tuning involves customizing pre-trained models for specific tasks. This process includes additional training on specialized datasets to ensure the model adapts to the new requirements. By focusing on these specific datasets, the model becomes more accurate and effective for particular applications. This tailored approach allows for better performance and precision in the given task.
9. Natural Language Processing (NLP)
Natural Language Processing (NLP) acts as the bridge between human language and computer understanding. By utilizing NLP, computers can process and analyze human language. This technology allows machines to interpret and respond to text and speech in ways that are useful and meaningful. Through NLP, computers can understand context, sentiment, and the nuances of human communication, making interactions between humans and machines more intuitive and effective.
10. Chatbots
Chatbots are AI conversational partners. They simulate human-like chat experiences by understanding and responding to user inputs. Through natural language processing (NLP), AI chatbot solutions can interpret various queries and provide relevant answers. This interaction creates a seamless and engaging experience for users, making communication with technology more intuitive and efficient.
11. OpenAI
OpenAI is the innovative lab responsible for creating groundbreaking AI models, including GPT-3 and DALL-E. These models have gained widespread recognition for their advanced capabilities. For instance, GPT-3 excels in generating human-like text, making it useful for various applications such as content creation and customer service. On the other hand, DALL-E is known for its unique ability to generate stunning images from textual descriptions, opening up new possibilities in the fields of art and design. By continually developing and refining these models, OpenAI developments contributes to the advancement of new AI and its integration into everyday applications.
12. LangChain
LangChain is a toolkit designed to integrate various AI models seamlessly. Moreover, it enhances applications by incorporating large language models (LLMs). This combination allows for more efficient and sophisticated AI solutions, making it easier to develop and deploy advanced AI applications.
13. Supervised Learning
Supervised learning trains AI with labeled data. In this process, the answers are already known, allowing the AI to learn by example. By providing a clear set of inputs and corresponding outputs, the system can effectively understand patterns and relationships within the data. This method ensures that the AI can make accurate predictions and decisions based on the examples it has been trained on.
14. Unsupervised Learning
Unsupervised learning allows AI to learn from data without predefined answers. By analyzing the data independently, it uncovers hidden patterns. This method helps AI find relationships and structures within the data that might not be immediately apparent. Consequently, it provides deeper insights and can identify trends that were previously unnoticed.
15. Reinforcement Learning
Reinforcement learning involves AI learning through trial and error. By continuously interacting with its environment, the AI receives feedback on its actions. This feedback helps the AI to refine its strategies and make better decisions over time. Through this iterative process, the AI becomes more adept at solving problems and achieving its goals.
16. Bias in AI
Bias in AI is a crucial issue to address. When data used to train AI models is biased, it can lead to skewed results. This can impact the fairness and accuracy of AI systems. For example, if an AI system is trained on data that underrepresents certain groups, it may perform poorly for those groups. Therefore, ensuring that training data is diverse and representative is essential. Additionally, ongoing monitoring and evaluation can help identify and mitigate bias in AI systems. By addressing these concerns, we can work towards more fair and accurate AI outcomes.
17. Ethics in AI
Ethics in AI serves as the moral compass for this technology. It guides how AI impacts our society and ensures that its development and application align with our values. Additionally, ethics in AI addresses concerns about fairness, transparency, and accountability. This helps prevent misuse and promotes trust in AI systems. Furthermore, ethical considerations in AI are crucial for protecting privacy and preventing discrimination. By focusing on these principles, we can develop AI technologies that benefit everyone and contribute positively to our world.
18. Explainable AI (XAI)
Explainable AI (XAI) enhances the transparency and understandability of AI’s decision-making processes. By providing clear insights into how AI systems reach their conclusions, XAI helps users trust and effectively use AI technology. This approach ensures that AI operations are not a mystery but are instead clear and comprehensible. Additionally, XAI supports better debugging and improvement of AI models, leading to more reliable and accurate outcomes.
19. GPT-3/GPT-4
GPT-3 and GPT-4 are iterations of OpenAI’s Generative Pre-trained Transformer. These models are widely recognized for their ability to mimic human-like text. By leveraging vast datasets, they can generate coherent and contextually relevant responses. As a result, they have found applications in diverse fields, such as customer service, content creation, and more. Their advanced language processing capabilities allow for natural and engaging interactions, making them valuable tools for businesses and individuals alike.
20. DALL-E
DALL-E, developed by OpenAI, is an innovative model that can transform written text into visually stunning images. This advanced AI technology allows users to generate creative and unique artwork by simply describing what they want to see. For instance, if you input a description of a “blue elephant standing in a field of sunflowers,” DALL-E will produce a vivid and detailed image that matches your description. This capability opens up new possibilities for artists, designers, and anyone interested in exploring the creative potential of AI.
Conclusion to AI Terms Guide
AI advancements are fascinating. The future is here and coded in these essential AI terms. By understanding these concepts, we can better appreciate how AI is changing our world. Moreover, these terms provide a foundation for exploring more advanced AI applications in various fields.
Feel free to share and spread the AI wisdom with friends and colleagues. As we continue to learn and grow together in this digital era, we can all stay informed and ready for the innovations that lie ahead.