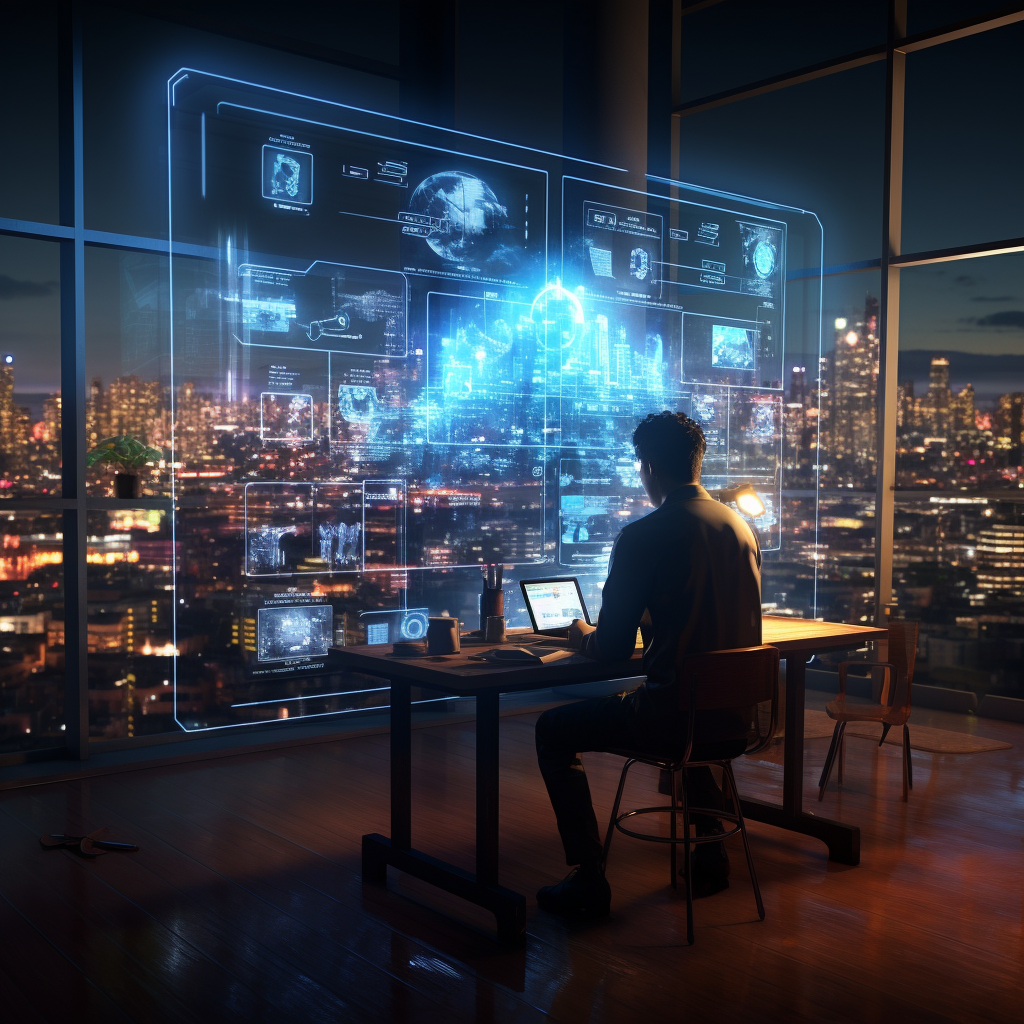
Fine-Tuning vs RAG: What`s Better for Your AI Project?
Fine-Tuning vs RAG: A Battle With Just One Winner?
Well, the “Fine-Tuning vs RAG” isn’t about picking a winner in a showdown. In the emerging AI field, selecting the right approach for optimizing AI models is crucial for developers and businesses alike. As it happens, two main methods in this area are LLM Fine-Tuning and RAG. Each has its pros and cons. So, let’s take a look where it shines the brightest.
So, in this guide, we’re diving deep into a comparison designed to inform our audience about the ins and outs of AI modeling. This friendly exploration aims to demystify the process and provide clear insights into how AI can work best for you and your business.
What is a RAG?
Retrieval-augmented generation (RAG) represents an innovative approach in the generative AI field. RAG integrates the capabilities of neural network models with the wealth of information stored in external knowledge bases. In other words, this method operates by first searching through a comprehensive database to find information relevant to the query at hand. Once AI retrieves relevant data, RAG uses this context to produce responses that are closely tailored to the query’s specifics.
This process allows RAG to address a broad spectrum of questions and topics, leveraging the extensive storage of knowledge available in various databases. The strength of RAG lies in its ability to pull from diverse sources, ensuring that the generated responses benefit from a rich informational foundation. As a result, RAG systems give detailed and knowledgeable answers in many areas without the expensive training that usually comes with creating new neural network models from the beginning.
Furthermore, RAG’s methodology facilitates a dynamic and adaptable AI solution. As the information sources RAG uses are updated and grown, its ability to provide relevant answers gets better. This inherent flexibility makes RAG an attractive option for applications that require access to the latest information or cover topics with fast-changing content.
RAG Application Benefits
Cost Efficiency
RAG’s approach to integrating external knowledge bases for generating responses lowers the financial barriers associated with deploying AI solutions. It saves a lot of money by using existing data instead of making and labeling huge new datasets to train advanced AI models from the beginning. This economical aspect of RAG makes it accessible for a wider range of projects, including those with limited budgets.
Quick Deployment
The framework of RAG facilitates the rapid development and launch of AI-driven applications. It minimizes the time-consuming process of training models on specific datasets by using already available external knowledge. This efficiency greatly benefits projects with tight deadlines or those that need to quickly adapt to market changes. As a result, businesses can deploy responsive AI solutions without the lengthy preparation phase that custom training needs.
Versatility for Standard Queries
One of the standout features of RAG is its adeptness at managing a broad spectrum of general information requests. By querying expansive knowledge bases, RAG is capable of sourcing and delivering accurate answers to a wide variety of standard queries. This versatility makes it an ideal choice for applications such as customer service bots, informational tools, or any AI application where the primary function is to provide factual, general knowledge. Its ability to tap into a wealth of information allows users receive relevant, precise responses, enhancing UX across diverse platforms.
RAG Deficiencies
Limited Customization
RAG relies heavily on information from existing knowledge bases to generate responses. This reliance restricts the model’s ability to produce answers that are highly tailored to unique questions. In scenarios where specificity and detailed customization are essential, RAG might not meet the required expectations, as it cannot deviate much from the information it retrieves.
Generalized Responses
Although RAG performs well with broad queries by sourcing accurate data from its extensive knowledge base. It may not achieve the same level of success with more specialized topics. When users seek in-depth information on a niche subject, RAG’s responses may appear too generalized. Consequently, it causes a lack of the detailed context that a more tailored approach could provide.
Adaptability to New Information
One of the inherent deficiencies of RAG is its limited ability to adapt to new information promptly. RAG models rely on established knowledge bases and might not show the latest trends unless those databases get regular updates. This delay in incorporating new insights can be a drawback in rapidly changing fields or situations where up-to-date information is critical for accurate response generation.
RAG Use Cases
FAQ Systems
RAG is well-suited for creating FAQ systems that can auto-generate responses to common queries across multiple domains. This capability allows for immediate, accurate answers to user questions, enhancing user experience and reducing the workload on staff.
Content Recommendation
RAG can analyze user preferences and past interactions to recommend articles, products, or services. This personalized approach encourages discovery and engagement, guiding users to items they are likely to find attractive.
Educational Tools and Learning Platforms
By integrating RAG into educational platforms, developers can provide students with instant access to information and explanations on diverse topics. This application supports personalized learning experiences, allowing students to explore subjects at their own pace and based on their interests.
Search Engines
RAG can improve the relevance and accuracy of search engine results. By drawing from extensive knowledge bases, RAG can provide users with answers that are based on the search query and enriched with additional context. Which makes information retrieval more efficient and user-friendly.
Interactive Storytelling and Gaming
In the realm of entertainment, RAG can create dynamic storytelling experiences and interactive games. It can generate narrative content in response to user choices or actions. Thus leading to personalized storylines and game experiences that adapt based on player interaction.
What is Fine-tuning LLM?
Fine-tuning LLM (Large Language Model) is like giving an AI model extra lessons. So it can get good at a specific job. Imagine you have a general-purpose robot that knows a little bit about everything. Fine-tuning is when you teach this robot more about a certain area, like cooking or gardening, so it becomes an expert in that field. You can do this by giving it more information and examples about the specific topic it needs to learn.
This process starts with a model that already knows a lot from previous training on a wide range of topics. Then, developers give it more data related to the specific task at hand. For example, if you want the AI to understand medical texts better, you would train it further with a lot of medical books, articles, and journals. This extra training helps the AI to understand the special words, concepts, and information that are important for medical discussions. It’s like tutoring the AI in a subject it needs to know more about.
Fine-tuning makes these AI models more useful and accurate for special tasks. After this additional training, the AI can answer questions, write, or analyze information in its area of expertise much better than before. This doesn’t mean it forgets what it learned before; it just adds more knowledge to its base. So, fine-tuning helps in making these AI models not just jack-of-all-trades but also masters of specific ones, improving how they perform specific tasks they’re tuned for.
Fine Tuning LLM Benefits
Highly Customized Solutions
Fine-tuning adjusts AI models to grasp the special language and intricate ideas of different industries. This means it can give answers that fit exactly what you’re looking for. Think of it like teaching a smart robot to speak the language of your specific business, ensuring it understands and responds in ways that are directly relevant to your needs.
Increased Accuracy
Accuracy is super important in areas where getting things right can’t be compromised, like in medicine or law. Fine-tuning polishes the AI model to make sure it gets its facts straight, answering questions or solving problems with a high level of precision. It’s like fine-tuning a musical instrument to hit the right notes every time.
Reflects Brand Voice
Every brand has its tone of voice. Fine-tuning helps AI models catch on to this unique style, making sure the way they communicate fits the brand’s personality. Whether your brand is friendly and casual or serious and professional, fine-tuning helps AI speak your language, making interactions with customers feel more natural and aligned with your brand’s identity.
Fine-Tuning LLM Deficiencies
Higher Costs
Fine-tuning an AI model is like teaching it a new skill, and it needs the right kind of data to learn properly. This means you have to find and prepare special data sets just for this purpose. But here’s the catch: this process can become pricey. It requires a lot of computer power and time from experts to adjust the model just right for what you need. So, while it’s a powerful approach, it also ends up being more expensive.
Time-Consuming
Fine-tuning takes time. It’s not just about the initial setup. You need to make careful adjustments and train the AI over and over to make sure it really gets what it needs to do. This means you have to wait a bit longer to get your AI up and running, especially compared to RAG. RAG is quicker to set up and start working. So, with fine-tuning, you’re looking at a slower start, but it’s all about getting the AI to perform just right.
Expertise Required
To properly fine-tune an AI model, you need experts in the field. These aren’t just any developers; they’re specialists who grasp the fine points of AI training. They know how to tweak a model to do exactly what you need. However, finding and hiring these experts is tough. It adds a whole new challenge to the process.
Fine-Tuning LLM Use Cases
Specialized Customer Service
In areas like healthcare or finance, customers often have complex questions that need precise answers. Fine-tuning allows AI to understand the specific language and needs of these sectors, providing answers that are both accurate and relevant. This means customers get the help they need quickly, making their experience much better. In this case, it’s best to develop an AI chatbot that will become a full-fledged business assistant on your website and increase conversions.
Custom Content Creation
When a brand wants to talk to its customers, the tone and style need to match the brand’s image. Fine-tuning helps AI learn how to create content that sounds like it comes directly from the brand, whether it’s blog posts, social media updates, or marketing emails. This way, the content feels more personal and engaging to the audience.
Enhanced Product Recommendations
For businesses that sell products or services, fine-tuning can make AI smarter about what customers might like. By understanding past shopping behavior and preferences, the AI can suggest items that the customer is more likely to buy. This sollution not only generates AI-based product recommendations for the customers but also helps businesses increase their sales.
Language Translation Services
In today’s global market, speaking the customer’s language is key. By improving AI models for translation services, businesses can provide precise and smooth translations. This improvement helps them reach more people while keeping the original message clear.
Predictive Maintenance
In industries such as manufacturing or transportation, machines are essential. When these machines stop working, it costs a lot of time and money. Luckily, we can use AI to help avoid these costly downtimes. AI works by looking closely at data from the machines. Then, it predicts when a machine might break down or need some upkeep. This way, AI can warn businesses early. So, they can repair the machine before it causes any disruptions. This smart approach saves both time and money, keeping everything running smoothly.
Fine-tuning vs RAG: Simplified and Clear Comparison
Choosing between fine-tuning your AI model or using RAG (Retrieval-Augmented Generation) depends a lot on what you need from your project. Let’s break it down to make it easier to understand.
When You Might Go for RAG:
RAG is like a smart assistant that uses a big library of information (knowledge base) to find and give you the answers you need. It’s great for:
- General Questions: When you just need answers to common questions, RAG can quickly pull the right info from its library.
- Saving Money: Since RAG uses information that’s already out there, it’s cheaper than having to teach your AI everything from scratch.
- Getting Started Fast: If you need to get your AI up and running quickly, RAG can be set up in no time.
When Fine-Tuning is the Way to Go:
Fine-tuning is more about teaching your AI specific tricks for your specific needs. It’s perfect for:
- Special Topics: If your project deals with unique topics or industry-specific language, you’ll want to fine-tune your AI to understand all of that correctly.
- Custom Answers: For responses that need to sound like they’re coming from your brand or need to be very specific, teaching your AI through fine-tuning is necessary.
- Accuracy is Key: In areas where every detail matters, like in healthcare or law, fine-tuning helps your AI give the right answers every time.
AI isn’t just one tool. It’s more like a whole set of tools where you need to pick the right one for the job. To compare Fine-tuning vs RAG it’s essential to grasp the main differences between its designations. Hopefully, this article helped you to make the best choice for your project.
Wrapping Up: Softblues AI is your top-tier AI Development Partner
If you’re considering generative AI integration services but are unsure about selecting the right AI model, we’re here to help. Click the button below to chat with Lumia, our AI-powered assistant, who can answer your questions and guide you through the options.
Our team at SoftBlues AI Agency is dedicated to providing custom AI solutions that meet your specific needs. Whether you’re in healthcare, fintech, e-commerce, retail, or manufacturing, our experts are ready to assist you in harnessing the full potential of AI.
Don’t miss this opportunity to enhance your business with cutting-edge AI technology. Start a conversation with our AI assistant today to explore how we can support your goals.
Click the button now to get started!