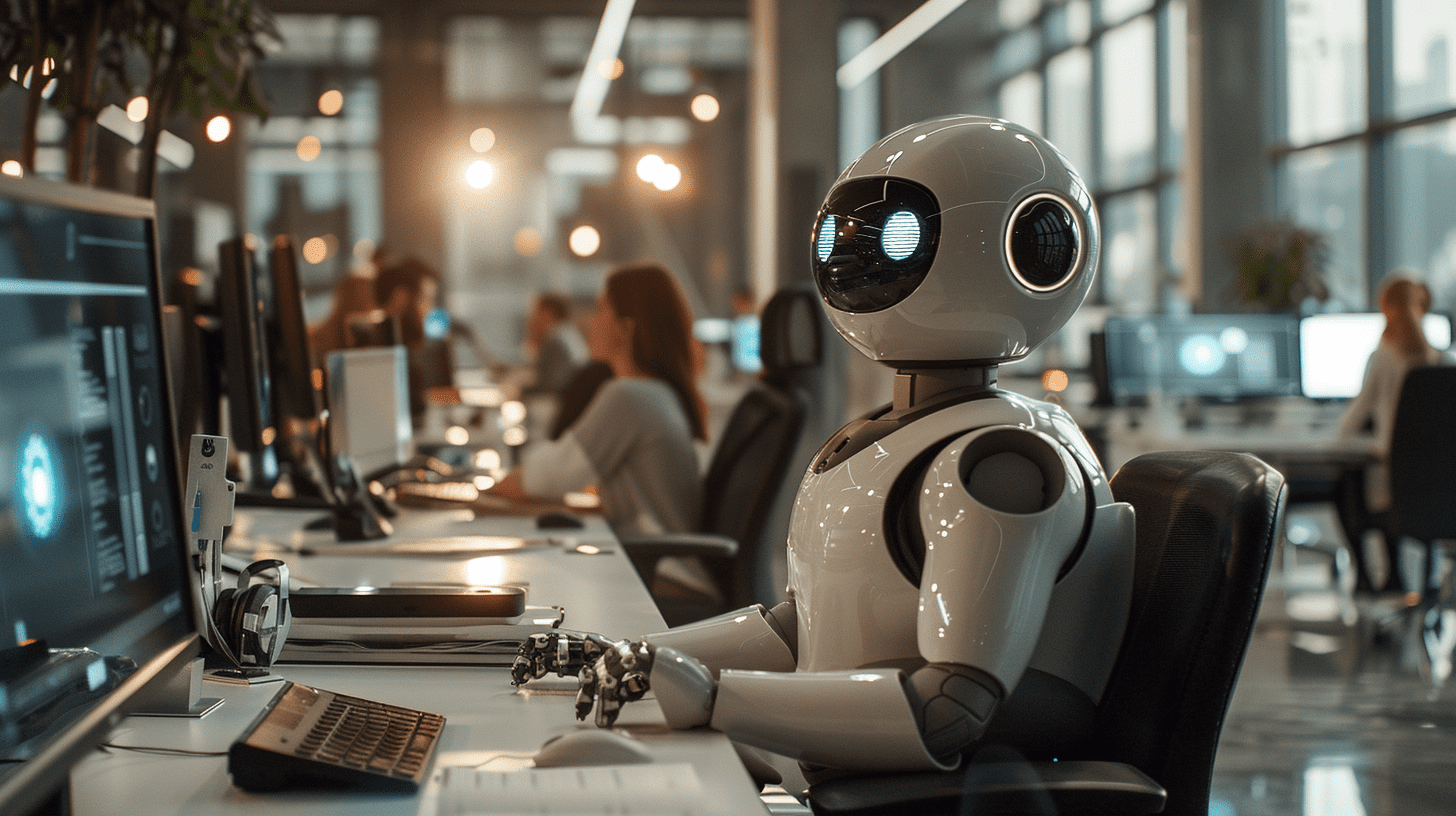
What is RAG in AI? The Key to Smarter Virtual Assistants
AI has made tremendous strides in assisting us with various tasks. One particularly interesting development is Retrieval-Augmented Generation (RAG). But what exactly is RAG, and why is it important? This article delves into the basics of RAG, its components, benefits, and potential for future applications.
What is RAG?
RAG, or Retrieval-Augmented Generation, is an advanced AI technique designed to improve the intelligence and functionality of chatbots and virtual assistants. It works by enabling these systems to search for and utilize information from trusted sources while responding to queries. This approach ensures that the answers provided are more precise and relevant, enhancing the overall usefulness and reliability of your interactions with AI. By incorporating external data into their responses, chatbots, AI assistants or multi-agent AI consultants can deliver information that is both current and accurate.
Why Does Retrieval-Augmented Generation Matter?
Accurate Answers
Retrieval-Augmented Generation guarantees that the information you receive is accurate. It achieves this by combining the power of large language models with up-to-date information retrieved from various sources. This approach not only provides comprehensive responses but also ensures they are based on the most current and relevant data available. By leveraging real-time information retrieval, RAG enhances the reliability and depth of the answers, making them both precise and informative.
Relevance
The responses are highly relevant to your specific questions. Each answer addresses the nuances of your queries, ensuring that you receive information that is both precise and useful. By focusing on the details you care about, the responses provide clarity and insight, making it easier for you to understand and apply the information in your context.
Trusted Sources
RAG gathers data from reliable, fact-checked sources to provide accuracy and credibility. This thorough vetting process helps build trust and confidence in the information provided. Reliable sources include peer-reviewed journals, reputable news organizations, academic institutions, and expert opinions. Each piece of information is carefully cross-verified with multiple sources to avoid any inaccuracies or biases. This meticulous approach ensures that the content is not only informative but also trustworthy, providing a solid foundation for making informed decisions.
Think of having a virtual assistant who can quickly search through a vast library of knowledge to provide the most helpful and dependable answer every time you ask a question. This capability is what makes RAG so powerful and essential.
Components of RAG
RAG is built around two main components, each playing a vital role in providing accurate and relevant information:
The Searcher
The searcher dives into a collection of trusted sources to find the most pertinent pieces of information for your query. It meticulously combs through available data, ensuring that what it retrieves is both reliable and relevant to the task at hand.
The Responder
Once the searcher has gathered the necessary information, the responder steps in. This component takes the collected data and integrates it with your original question. It then crafts a comprehensive and informed response, tailored to address your specific needs effectively.
Benefits of RAG
- Trustworthy Answers: The answers provided by RAG are supported by reliable sources, ensuring you receive accurate and dependable information.
- Transparency: With RAG, you can see the sources of the information, which builds trust and confidence in the responses.
- Smarter Responses: RAG enhances AI systems by providing more accurate and useful answers. It searches for information to ensure the responses are well-informed and relevant.
- Improved User Experience: By delivering precise and reliable answers, RAG enhances the overall user experience, making interactions with AI systems more satisfying.
- Enhanced Decision-Making: Access to reliable and transparent information allows users to make better-informed decisions, whether in personal use or professional contexts.
- Efficiency in Information Retrieval: RAG quickly searches and retrieves relevant information, saving users time and effort compared to manual searches.
- Consistency in Responses: RAG ensures that the information provided remains consistent and up-to-date, which is crucial for maintaining the integrity of AI systems.
Discuss Your Insights
RAG (Retrieval-Augmented Generation) is becoming a hot topic in the AI community. This advanced technique combines the strengths of retrieval-based systems and generation-based models, promising to enhance the accuracy and relevance of AI-generated content. By integrating external data sources into the generation process, RAG can provide more precise and contextually relevant responses.
We’d love to hear your thoughts on this emerging technology. How do you envision RAG shaping the future of AI applications? What potential benefits or challenges do you foresee? Share your insights and join the conversation in the comments below.